Geometry Objects: Representation, Parametrization, and Mapping#
Why geometries in CUQIpy?#
Geometry objects in CUQIpy serve two main purposes:
They simplify visualization by embedding plotting tools directly within the arrays and objects, such as
CUQIArrays
andSamples
. This is demonstrated throughout the book using methods likeplot
.They provide a mapping between parameter vectors and function values, enabling CUQIpy’s sampling algorithms (operating on vectors) to be compatible with more complex representations like 2D images.
Parametrization and Mapping with Geometries#
One way to view the Geometry object is as a parameter-to-function mapping (and sometimes also a function-to-parameter mapping). The function space is what the forward models are operating on, e.g. a 2D convolution on images, while the parameter space is what the sampling algorithms are operating on. These mappings can also be more complicated, such as a mapping coefficients of an expansion to the function values on a PDE mesh.

A vector of values (unknown parameters or data) in the Bayesian Inverse problem setting can be interpreted in various ways. It can be, for example, a vector of function values at 1D or 2D grid points, a vector of image pixels, a vector of expansion coefficients, or a collection of variables, e.g. the temperature measurement at four cities: A, B, C, and D.
In CUQIpy, the cuqi.geometry
module provides classes for different representations of vectors, e.g Continuous1D
, Continuous2D
, Image2D
, and Discrete
.
Here we explore geometry assignment for Distributions
, Samples
and CUQIarray
. We explore the three main functionalities of the geometry objects: representation, parametrization, and mapping.
Variable representation #
First let us create 1000 samples from a 4-element distribution \(y\):
Show code cell content
from cuqi.distribution import Gaussian
from cuqi.geometry import Image2D, Discrete, StepExpansion, MappedGeometry
from cuqi.array import CUQIarray
import numpy as np
mu = np.array([5, 3, 1, 0])
sigma = np.array([1, 2, 3, 0.5])
y = Gaussian(mean=mu, cov=sigma**2)
y_samples = y.sample(1000)
If no geometry is provided by the user, when creating a Distribution
for example, CUQIpy will assign _DefaultGeometry1D
(trivial geometry) to the distribution and the Samples
produced from it.
print(y.geometry)
print(y_samples.geometry)
_DefaultGeometry1D(4,)
_DefaultGeometry1D(4,)
Samples are plotted with line plot by default, which is due to how the _DefaultGeometry1D
interprets the samples.
y_samples.plot([100,200,300])
[<matplotlib.lines.Line2D at 0x7fc5a1cddc30>,
<matplotlib.lines.Line2D at 0x7fc5a1cddd20>,
<matplotlib.lines.Line2D at 0x7fc5a1cdde10>]
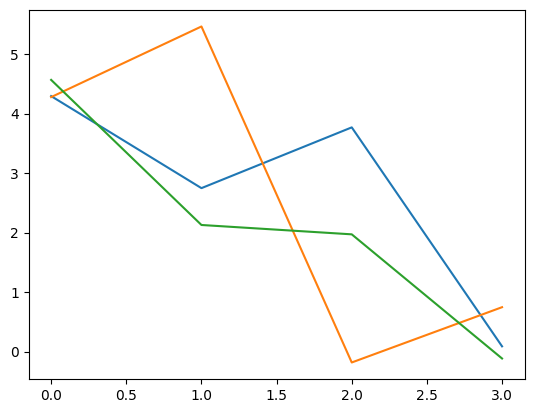
We may equip the distribution with a different geometry, either when creating it, or afterwards. Let us for example assign an Image2D
geometry to the distribution y
. First we create the Image2D
geometry and we assume the shape of the image is \(2\times2\):
geom_image = Image2D((2,2))
print(geom_image)
Image2D(4,)
We can check the number of parameters (parameter dimension) of the geometry:
geom_image.par_dim
4
We can also check the shape of its representation (size of the image in this case) using the property fun_shape
:
geom_image.fun_shape
(2, 2)
Now we equip the distribution y
with this Image2D
geometry.
y.geometry = geom_image
Check the geometry of y
:
y.geometry
Image2D(4,)
With this distribution set up, we are ready to generate some samples
# call method to sample
y_samples = y.sample(50)
We can check that we have produced 50 samples, each of size 4:
y_samples.shape
(4, 50)
We plot a couple of samples:
y_samples.plot()
Plotting 5 randomly selected samples
[<matplotlib.image.AxesImage at 0x7fc5a1d99420>,
<matplotlib.image.AxesImage at 0x7fc5a1dd2710>,
<matplotlib.image.AxesImage at 0x7fc59fc3d9c0>,
<matplotlib.image.AxesImage at 0x7fc59fc3f310>,
<matplotlib.image.AxesImage at 0x7fc59fca7280>]
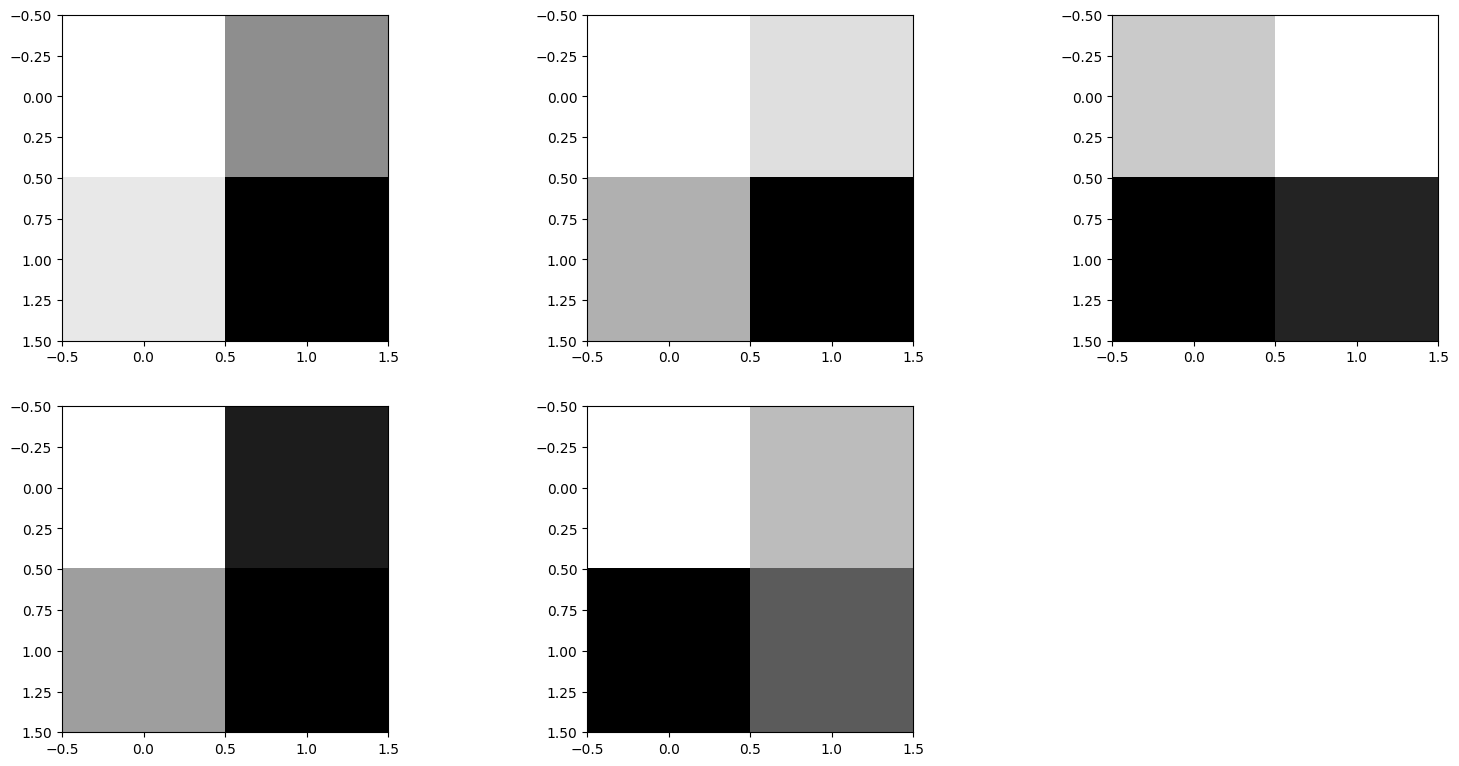
What if the 4 parameters in samples have a different meaning? For example, the four parameters might represent labelled quantities such as temperature measurement at four cities A, B, C, D. In this case, we can use a Discrete
geometry:
geom_discrete = Discrete(['Temperature A', 'Temperature B', 'Temperature C', 'Temperature D'])
print(geom_discrete)
Discrete(4,)
We can update the distribution’s geometry and generate some new samples:
y.geometry = geom_discrete
y_samples = y.sample(100)
The samples will now know about their new Discrete
geometry and the plotting style will be changed:
y_samples.plot();
Plotting 5 randomly selected samples
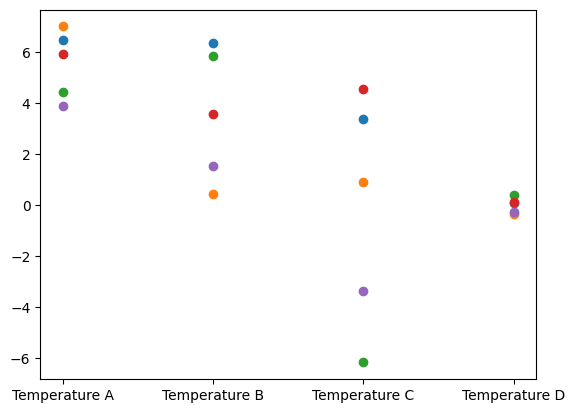
The credibility interval plot style is also updated to show errorbars for the Discrete
geometry:
y_samples.plot_ci(95, exact=mu)
[<matplotlib.lines.Line2D at 0x7fc59efdefe0>,
<matplotlib.lines.Line2D at 0x7fc59f0009a0>,
<ErrorbarContainer object of 3 artists>]
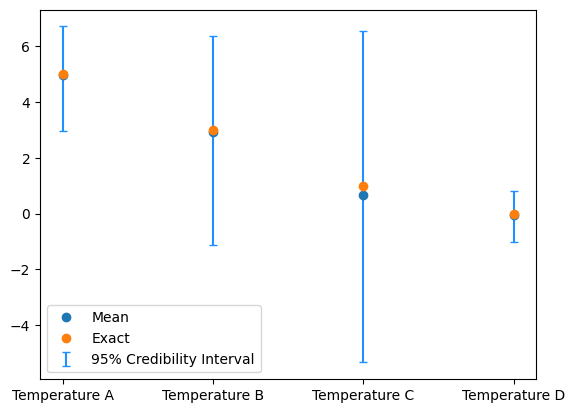
And similarly, in the chain plot, the legend reflects the particular labels:
y_samples.plot_chain([2,0])
[<matplotlib.lines.Line2D at 0x7fc59fa099f0>,
<matplotlib.lines.Line2D at 0x7fc59fa09ae0>]
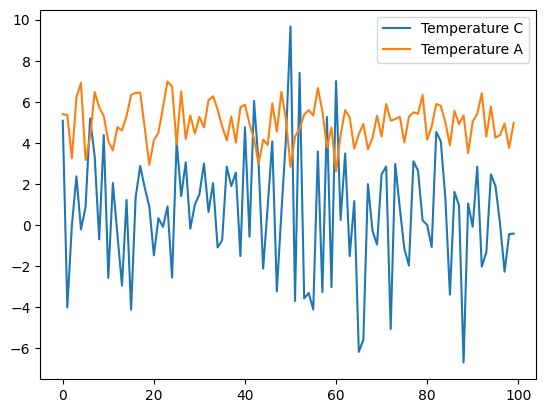
Varible parameterization #
In CUQIpy we have geometries that represents a particular parameterization of the variables (e.g. StepExpansion
, KLExpansion
, etc).
For example, in the StepExpansion
geometry, the parameters represent expansion coefficients for equidistance-step basis functions. You can read more about StepExpansion
by typing help(cuqi.geometry.StepExpansion)
in a the code cell below or by looking at the documentation of StepExpansion
Let us create a StepExpansion
geometry, we first need to create a grid on which the step functions are defined, let us assume the grid is defined on the interval \([0,1]\):
grid = np.linspace(0, 1, 100)
Then we create the StepExpansion
geometry with 4 steps and assign it to the distribution y
:
geom_step_expantion = StepExpansion(grid, n_steps=4)
y.geometry = geom_step_expantion
print(y.geometry)
StepExpansion(4,)
Let us samples the distribution y
and plot the samples:
y_step_samples = y.sample(100)
y_step_samples.plot()
Plotting 5 randomly selected samples
[<matplotlib.lines.Line2D at 0x7fc59fa93250>,
<matplotlib.lines.Line2D at 0x7fc59fa93340>,
<matplotlib.lines.Line2D at 0x7fc59fa93430>,
<matplotlib.lines.Line2D at 0x7fc59fa93520>,
<matplotlib.lines.Line2D at 0x7fc59fa920b0>]
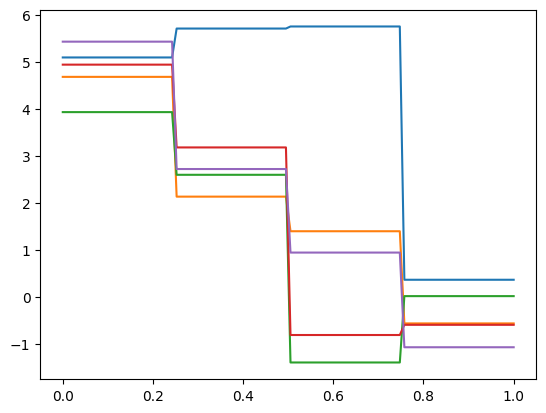
Note that the samples are now represented as expansion coefficients of the step functions.
Examples of using StepExpansion
and KLExpansion
geometries in the context of a PDE parameterization for a heat 1D BIP can be found here, and here [ARA+24], respectively.
Variable mapping #
In CUQIpy
, we provide the MappedGeometry
object which equips geometries with a mapping function that are applied to the variables, this mapping can also be viewed as parametrization. An example of a commonly used mapping in inverse problems is \(e^x\) for unknown parameters \(x\) to ensure positivity regardless of the value of \(x\).
Let us use the MappedGeometry
to map the StepExpansion
geometry we created earlier with the exponential function:
geom_step_expantion_mapped = MappedGeometry(geom_step_expantion, map=lambda x: np.exp(x))
Let us again, assign the MappedGeometry
to the distribution y
and generate samples and plot them:
y.geometry = geom_step_expantion_mapped
y_mapped_step_samples = y.sample(100)
y_mapped_step_samples.plot()
Plotting 5 randomly selected samples
[<matplotlib.lines.Line2D at 0x7fc59f92a3e0>,
<matplotlib.lines.Line2D at 0x7fc59f92a4d0>,
<matplotlib.lines.Line2D at 0x7fc59f92a5c0>,
<matplotlib.lines.Line2D at 0x7fc59f92a6b0>,
<matplotlib.lines.Line2D at 0x7fc59f92a7a0>]
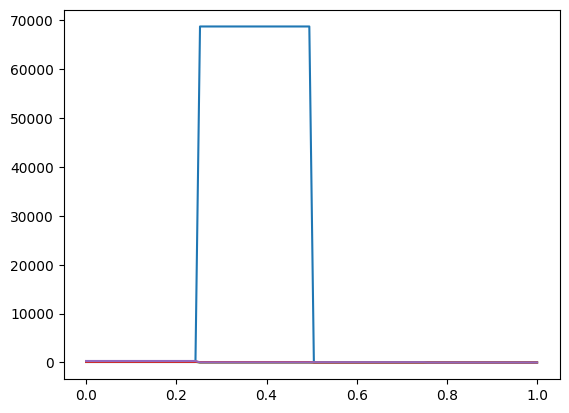
Note that the samples are still representing the expansion coefficients of the step functions, but the mapping function \(e^x\) has been applied to the samples. All samples are now non-negative.
★ Elaboration: Specifying a Geometry object for CUQIarray objects:#
Geometries can also be specified for CUQIarray
, the basic array structure in CUQIpy. Let us create a CUQIarray
object with four parameters as follows:
q = CUQIarray([1,5,6,0])
We look at the geometry property
q.geometry
_DefaultGeometry1D(4,)
And then let us plot our variable q
:
q.plot()
[<matplotlib.lines.Line2D at 0x7fc59f9b9d50>]
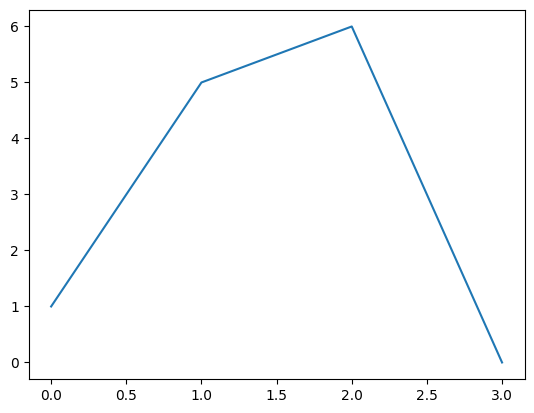
We now choose a different interpretation for the variable q
by changing its geometry to, for example, the Image2D
geometry we created, and then we plot:
q.geometry = geom_image
q.plot()
[<matplotlib.image.AxesImage at 0x7fc59f803190>]
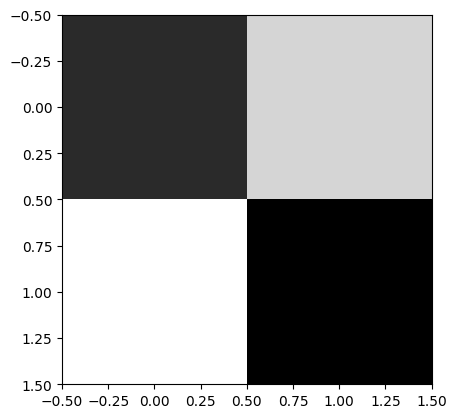
Finally we set the Discrete
geometry we created as the geometry for q
and plot:
q.geometry = geom_discrete
q.plot()
[<matplotlib.lines.Line2D at 0x7fc59f8c1960>]
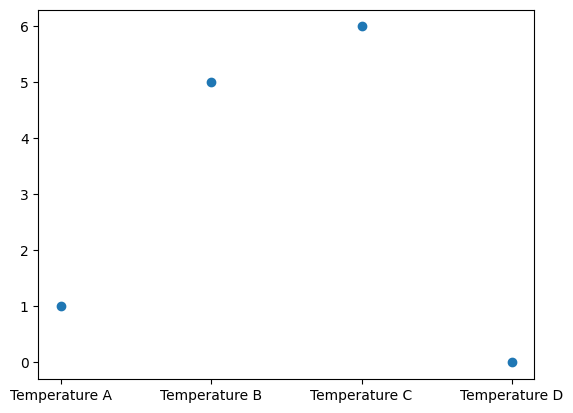